by Gloria Nobile
Einstein Telescope (ET), the future third-generation gravitational wave detector, will be one of the most important European research infrastructures of the coming decades. As an evolution of the current interferometers, Advanced LIGO, Advanced Virgo, and KAGRA, ET will make it possible to study a volume of the universe at least a thousand times larger, thanks to its significantly enhanced sensitivity, the increased size of the detector, and the implementation of new and innovative technologies.
Once the telescope is operational, a continuous stream of astrophysical signals is expected: dozens of events per day, each containing fundamental clues about the origin of the universe. This enormous amount of data will require increasingly fast and efficient analysis tools. For this reason, researchers are already exploring artificial intelligence (AI) techniques capable of quickly distinguishing the desired signal from sources of interference or noise that could compromise experimental measurements. One of the key figures in this effort is Elena Cuoco, professor at the University of Bologna, member of the Virgo and ET collaboration, and co-coordinator of the division developing a data analysis platform for ET. We interviewed her.
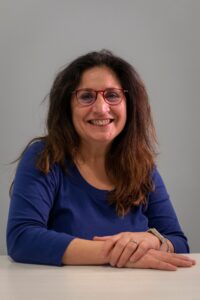
Elena Cuoco
You have contributed to the development of data analysis techniques, particularly to move from noise analysis to transient signal detection. What are they?
These are signals that last only a short time but are capable of releasing a huge amount of energy. They can be related to astrophysical phenomena, such as the coalescence of binary stars – which are exactly the signals we have detected – or the collapse of a massive star, like a supernova. But in our data, there are also transient signals due to noise. The technical term is “glitch”, meaning a an energy spike released in a very short time. It’s very important to be able to characterize them in order to remove them and isolate the real signal.
How?
We’re dealing with a very small astrophysical signal embedded in a very large noise. To clean the data from this noise, we try to model it. Specifically, artificial intelligence techniques can be used to identify and remove glitches.
Can you give us an example?
If you have a large number of glitches available, you can build a dataset with many examples and use it to train an AI algorithm. This algorithm learns to recognize the features of those signals, just like the algorithms Google uses to recognize our voice, face, or habits. Once trained, the algorithm can automatically recognize new glitches because it has already learned how to do it. It’s kind of like the learning process of children: they see something over and over again, and then they are able to recognize it when it comes up again.
So the AI has to “see” the data. But how is it represented?
In our case, it is a time series, similar to an electric signal that varies over time. We can transform these series into images using signal processing techniques. This way, a transient or glitch can be visually represented with an image that preserves its key features. That makes it easier for the algorithm to recognize it.
With ET, observations will be daily and the data to analyze will increase significantly. Will AI help speed up the process?
Exactly. When we start detecting dozens of astrophysical signals per day, it will be essential to quickly analyze both the signals and the noise. We are developing techniques that accelerate crucial steps, such as data quality assessment and noise characterization. The first filtering can already be done once the algorithm has learned to recognize the signals. With ET, we’ll have much more data, but also more unknown types of noise, so we’ll need to be as fast as possible. Personally, I can’t imagine analysis without the AI contribution at some level.
And what could be the risks?
Ah, the risks! This is an old topic: there’s always the fear that your algorithm might learn something wrong and make mistakes. Actually, these techniques are used for real-time analysis to extract information as quickly as possible, but the data is always retained. We can always go back and re-analyze it. In general, if the signals are very energetic, it is difficult to make a mistake. With weaker signals, it might happen, but there’s always time to repeat the analysis, even with different methods. Moreover, in recent years there’s been a lot of work on so-called “explainability”, the ability to understand what the algorithm has learned and whether its predictions are reproducible. I don’t see risks, but benefits. Obviously, those who build these algorithms must deeply understand the physics of their experiment. So first, you need to know how to do standard data analysis, then you can apply artificial intelligence.
You mention multimodal techniques applied to multi-messenger astronomy. Does AI fit into this approach?
Absolutely. The idea that I have been pursuing for some years and continue to promote is to use a global approach to data analysis, leveraging different inputs: time series, images, neutrinos, gamma-ray bursts… With an algorithm trained to recognize information from multimessenger events – which we expect with the Einstein Telescope – it will be possible to uncover new physics.
Down memory lane: you were part of the team that announced the discovery of gravitational waves to the world. What does that mean to you, and how do you feel today, with even more advanced tools at your disposal?
I feel very lucky to have been part of that team and to have experienced that moment live. I still remember when a colleague emailed us saying, “We’ve seen this strange thing.” I replied, “What are you talking about?”, because even for us, who had been working on it for years, it seemed unbelievable. It was an incredibly emotional moment. Today, we’re almost used to it; the excitement remains, but we’re always hoping to move toward new discoveries. First, it was the merger of two black holes, then of neutron stars. My hope is to detect a supernova. With ET, we’ll be able to explore new physics, getting closer and closer to the Big Bang. I’m sure we’re in for some amazing discoveries.
Featured image credit: Raúl Rubio/Virgo Valencia Group/Virgo collaboration.